Understanding the Inner Workings of Generative AI: A Comprehensive Guide
Generative AI, also known as generative adversarial networks (GANs), is a fascinating field that has gained significant attention in recent years. This technology has the ability to create new content, such as images, music, and even language, that is indistinguishable from what humans can produce. But how does generative AI work? In this comprehensive guide, we will delve into the inner workings of generative AI and explore its fundamental principles.
Introduction to Generative AI
Generative AI is a branch of artificial intelligence that focuses on creating new content rather than simply analyzing or classifying existing data. It employs a two-part system consisting of a generator and a discriminator. The generator generates new content based on patterns it learns from a training dataset, while the discriminator evaluates the generated content and provides feedback to improve future iterations.
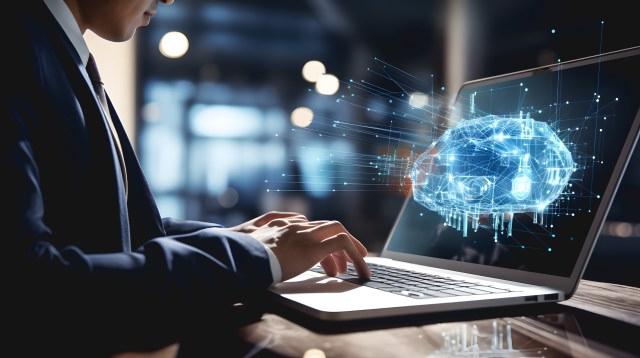
The Adversarial Training Process
The heart of generative AI lies in the adversarial training process. During this process, the generator and discriminator engage in an ongoing game where they try to outsmart each other. The generator’s goal is to create content that fools the discriminator into thinking it is real, while the discriminator’s goal is to accurately distinguish between real and generated content.
The training process begins with an initial random input for the generator. It generates a sample that is then evaluated by the discriminator. Based on this evaluation, both models are updated using backpropagation techniques to improve their performance. This iterative process continues until both models reach a point where the generated content is virtually indistinguishable from real data.
Understanding Neural Networks in Generative AI
Neural networks play a crucial role in generative AI by providing the foundation for both the generator and discriminator models. These networks consist of interconnected nodes or “neurons” that mimic how our own brains process information. The nodes are organized in layers, with each layer performing specific computations.
The generator network typically uses a type of neural network known as a deep convolutional generative adversarial network (DCGAN). This architecture allows the generator to learn complex patterns and generate high-quality content, such as realistic images. The discriminator network, on the other hand, is often a convolutional neural network (CNN) that specializes in image classification.
Real-World Applications and Future Possibilities
Generative AI has already made significant contributions to various fields. In the realm of art and design, it has been used to create unique paintings, music compositions, and even fashion designs. In healthcare, generative AI has shown promise in generating synthetic medical images for training and research purposes.
Looking ahead, the possibilities for generative AI are vast. It has the potential to revolutionize content creation by automating tasks that were previously done by humans. Imagine a world where AI-generated content is seamlessly integrated into our daily lives, from personalized advertisements to virtual reality experiences.
In conclusion, generative AI is an exciting field that combines advanced algorithms with neural networks to create new and realistic content. By understanding its inner workings – from the adversarial training process to the role of neural networks – we can appreciate its potential impact on various industries. As technology continues to advance, generative AI will undoubtedly play an increasingly important role in shaping our digital landscape.
This text was generated using a large language model, and select text has been reviewed and moderated for purposes such as readability.